تاريخ الرياضيات
الاعداد و نظريتها
تاريخ التحليل
تار يخ الجبر
الهندسة و التبلوجي
الرياضيات في الحضارات المختلفة
العربية
اليونانية
البابلية
الصينية
المايا
المصرية
الهندية
الرياضيات المتقطعة
المنطق
اسس الرياضيات
فلسفة الرياضيات
مواضيع عامة في المنطق
الجبر
الجبر الخطي
الجبر المجرد
الجبر البولياني
مواضيع عامة في الجبر
الضبابية
نظرية المجموعات
نظرية الزمر
نظرية الحلقات والحقول
نظرية الاعداد
نظرية الفئات
حساب المتجهات
المتتاليات-المتسلسلات
المصفوفات و نظريتها
المثلثات
الهندسة
الهندسة المستوية
الهندسة غير المستوية
مواضيع عامة في الهندسة
التفاضل و التكامل
المعادلات التفاضلية و التكاملية
معادلات تفاضلية
معادلات تكاملية
مواضيع عامة في المعادلات
التحليل
التحليل العددي
التحليل العقدي
التحليل الدالي
مواضيع عامة في التحليل
التحليل الحقيقي
التبلوجيا
نظرية الالعاب
الاحتمالات و الاحصاء
نظرية التحكم
بحوث العمليات
نظرية الكم
الشفرات
الرياضيات التطبيقية
نظريات ومبرهنات
علماء الرياضيات
500AD
500-1499
1000to1499
1500to1599
1600to1649
1650to1699
1700to1749
1750to1779
1780to1799
1800to1819
1820to1829
1830to1839
1840to1849
1850to1859
1860to1864
1865to1869
1870to1874
1875to1879
1880to1884
1885to1889
1890to1894
1895to1899
1900to1904
1905to1909
1910to1914
1915to1919
1920to1924
1925to1929
1930to1939
1940to the present
علماء الرياضيات
الرياضيات في العلوم الاخرى
بحوث و اطاريح جامعية
هل تعلم
طرائق التدريس
الرياضيات العامة
نظرية البيان
k-Statistic
المؤلف:
Carver, H. C. (Ed.)
المصدر:
"Fundamentals of the Theory of Sampling." Ann. Math. Stat. 1
الجزء والصفحة:
...
14-2-2021
2574
k-Statistic
The th
-statistic
is the unique symmetric unbiased estimator of the cumulant
of a given statistical distribution, i.e.,
is defined so that
![]() |
(1) |
where denotes the expectation value of
(Kenney and Keeping 1951, p. 189; Rose and Smith 2002, p. 256). In addition, the variance
![]() |
(2) |
is a minimum compared to all other unbiased estimators (Halmos 1946; Rose and Smith 2002, p. 256). Most authors (e.g., Kenney and Keeping 1951, 1962) use the notation for
-statistics, while Rose and Smith (2002) prefer
.
The -statistics can be given in terms of the sums of the
th powers of the data points as
![]() |
(3) |
then
![]() |
![]() |
![]() |
(4) |
![]() |
![]() |
![]() |
(5) |
![]() |
![]() |
![]() |
(6) |
![]() |
![]() |
![]() |
(7) |
(Fisher 1928; Rose and Smith 2002, p. 256). These can be given by KStatistic[r] in the Mathematica application package mathStatica.
For a sample size , the first few
-statistics are given by
![]() |
![]() |
![]() |
(8) |
![]() |
![]() |
![]() |
(9) |
![]() |
![]() |
![]() |
(10) |
![]() |
![]() |
![]() |
(11) |
where is the sample mean,
is the sample variance, and
is the
th sample central moment (Kenney and Keeping 1951, pp. 109-110, 163-165, and 189; Kenney and Keeping 1962).
The variances of the first few -statistics are given by
![]() |
![]() |
![]() |
(12) |
![]() |
![]() |
![]() |
(13) |
![]() |
![]() |
![]() |
(14) |
![]() |
![]() |
![]() |
(15) |
An unbiased estimator for is given by
![]() |
(16) |
(Kenney and Keeping 1951, p. 189). In the special case of a normal parent population, an unbiased estimator for is given by
![]() |
(17) |
(Kenney and Keeping 1951, pp. 189-190).
For a finite population, let a sample size be taken from a population size
. Then unbiased estimators
for the population mean
,
for the population variance
,
for the population skewness
, and
for the population kurtosis excess
are
![]() |
![]() |
![]() |
(18) |
![]() |
![]() |
![]() |
(19) |
![]() |
![]() |
![]() |
(20) |
![]() |
![]() |
![]() |
(21) |
(Church 1926, p. 357; Carver 1930; Irwin and Kendall 1944; Kenney and Keeping 1951, p. 143), where is the sample skewness and
is the sample kurtosis excess.
REFERENCES:
Carver, H. C. (Ed.). "Fundamentals of the Theory of Sampling." Ann. Math. Stat. 1, 101-121, 1930.
Church, A. E. R. "On the Means and Squared Standard-Deviations of Small Samples from Any Population." Biometrika 18, 321-394, 1926.
Fisher, R. A. "Moments and Product Moments of Sampling Distributions." Proc. London Math. Soc. 30, 199-238, 1928.
Halmos, P. R. "The Theory of Unbiased Estimation." Ann. Math. Stat. 17, 34-43, 1946.
Irwin, J. O. and Kendall, M. G. "Sampling Moments of Moments for a Finite Population." Ann. Eugenics 12, 138-142, 1944.
Kenney, J. F. and Keeping, E. S. Mathematics of Statistics, Pt. 2, 2nd ed. Princeton, NJ: Van Nostrand, 1951.
Kenney, J. F. and Keeping, E. S. "The -Statistics." §7.9 in Mathematics of Statistics, Pt. 1, 3rd ed. Princeton, NJ: Van Nostrand, pp. 99-100, 1962.
Rose, C. and Smith, M. D. "k-Statistics: Unbiased Estimators of Cumulants." §7.2C in Mathematical Statistics with Mathematica. New York: Springer-Verlag, pp. 256-259, 2002.
Stuart, A.; and Ord, J. K. Kendall's Advanced Theory of Statistics, Vol. 2A: Classical Inference & the Linear Model, 6th ed. New York: Oxford University Press, 1999.
Ziaud-Din, M. "Expression of the k-Statistics and
in Terms of Power Sums and Sample Moments." Ann. Math. Stat. 25, 800-803, 1954.
Ziaud-Din, M. "The Expression of -Statistic
in Terms of Power Sums and Sample Moments." Ann. Math. Stat. 30, 825-828, 1959.
Ziaud-Din, M. and Ahmad, M. "On the Expression of the -Statistic
in Terms of Power Sums and Sample Moments." Bull. Internat. Stat. Inst. 38, 635-640, 1960.
الاكثر قراءة في الاحتمالات و الاحصاء
اخر الاخبار
اخبار العتبة العباسية المقدسة
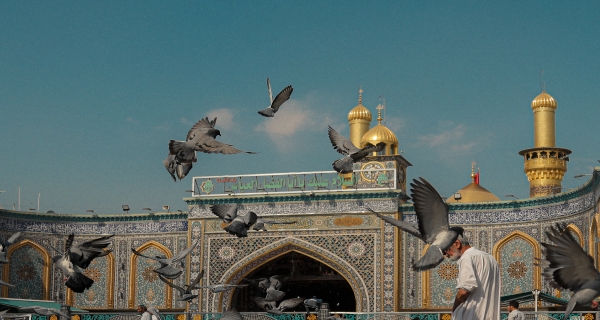
الآخبار الصحية
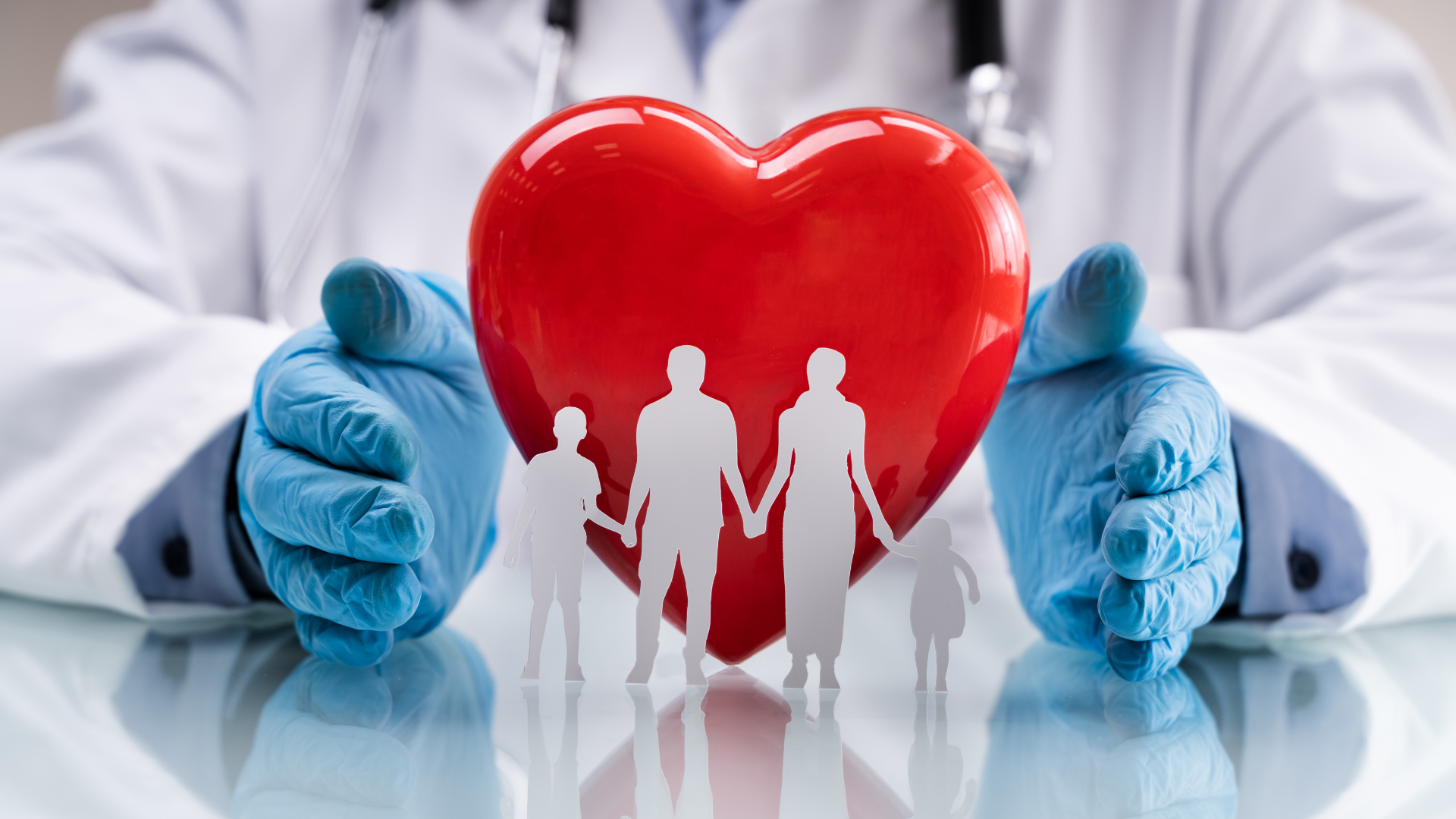