تاريخ الفيزياء
علماء الفيزياء
الفيزياء الكلاسيكية
الميكانيك
الديناميكا الحرارية
الكهربائية والمغناطيسية
الكهربائية
المغناطيسية
الكهرومغناطيسية
علم البصريات
تاريخ علم البصريات
الضوء
مواضيع عامة في علم البصريات
الصوت
الفيزياء الحديثة
النظرية النسبية
النظرية النسبية الخاصة
النظرية النسبية العامة
مواضيع عامة في النظرية النسبية
ميكانيكا الكم
الفيزياء الذرية
الفيزياء الجزيئية
الفيزياء النووية
مواضيع عامة في الفيزياء النووية
النشاط الاشعاعي
فيزياء الحالة الصلبة
الموصلات
أشباه الموصلات
العوازل
مواضيع عامة في الفيزياء الصلبة
فيزياء الجوامد
الليزر
أنواع الليزر
بعض تطبيقات الليزر
مواضيع عامة في الليزر
علم الفلك
تاريخ وعلماء علم الفلك
الثقوب السوداء
المجموعة الشمسية
الشمس
كوكب عطارد
كوكب الزهرة
كوكب الأرض
كوكب المريخ
كوكب المشتري
كوكب زحل
كوكب أورانوس
كوكب نبتون
كوكب بلوتو
القمر
كواكب ومواضيع اخرى
مواضيع عامة في علم الفلك
النجوم
البلازما
الألكترونيات
خواص المادة
الطاقة البديلة
الطاقة الشمسية
مواضيع عامة في الطاقة البديلة
المد والجزر
فيزياء الجسيمات
الفيزياء والعلوم الأخرى
الفيزياء الكيميائية
الفيزياء الرياضية
الفيزياء الحيوية
الفيزياء العامة
مواضيع عامة في الفيزياء
تجارب فيزيائية
مصطلحات وتعاريف فيزيائية
وحدات القياس الفيزيائية
طرائف الفيزياء
مواضيع اخرى
Joint and Conditional Entropies
المؤلف:
Garnett P. Williams
المصدر:
Chaos Theory Tamed
الجزء والصفحة:
331
16-3-2021
1352
Joint and Conditional Entropies
Joint and conditional entropies, as defined in this chapter and as used in information theory, apply to two systems or dimensions; for more than two dimensions (discussed later in the chapter), terminology is still developing. For simplicity, we'll usually deal here with two systems. The first, system X, has discrete observations xi; the second, system Y, has values yj.
The probability of seeing particular values of two or more variables in some sort of combination. In fact, we rolled a pair of dice (one white and one green, representing separate systems) and built a joint probability distribution. The joint probability distribution gave the probability of the joint occurrence of each possible combination of values of the two systems. Since entropy is based solely on probability, the idea of joint probability easily applies to a so-called joint entropy—the average amount of information obtained (or uncertainty reduced) by individual measurements of two or more systems.
Having built a word concept of joint entropy, let's follow our standard procedure and express it in symbols. There are two cases. In the first, knowing value x from system X has no effect on the entropy of system Y. (In other words, individual observations x and y aren't mutually related.) In the second, knowing x affects our estimate of the entropy of system Y (and so x and y have some kind of association). For each case, the symbols (and hence the form of the definition) can be either those of probabilities or of entropies.
Mutually unrelated systems
Let's begin by talking about the first case, in which x and y aren't mutually related. We can express joint entropy in terms of probabilities or in terms of entropies. First we'll do the probability form, then the entropy form.
الاكثر قراءة في مواضيع اخرى
اخر الاخبار
اخبار العتبة العباسية المقدسة
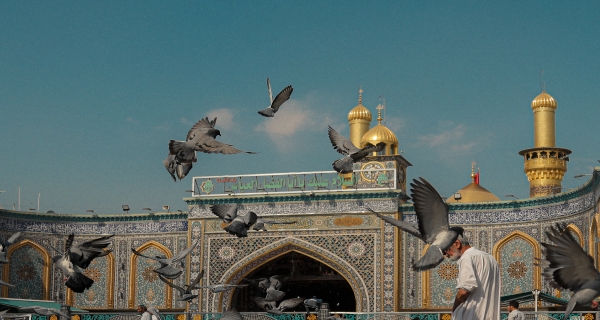
الآخبار الصحية
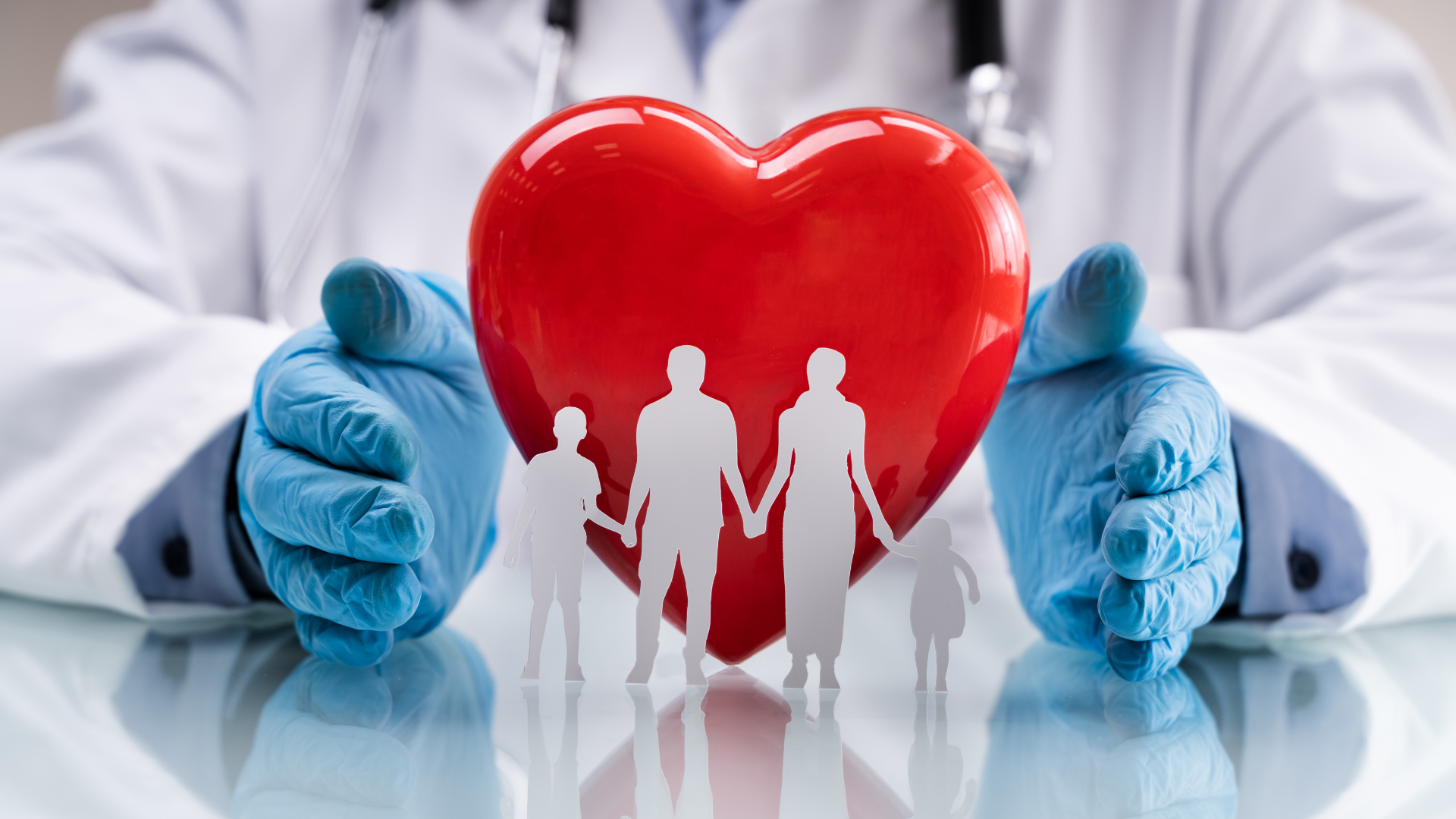